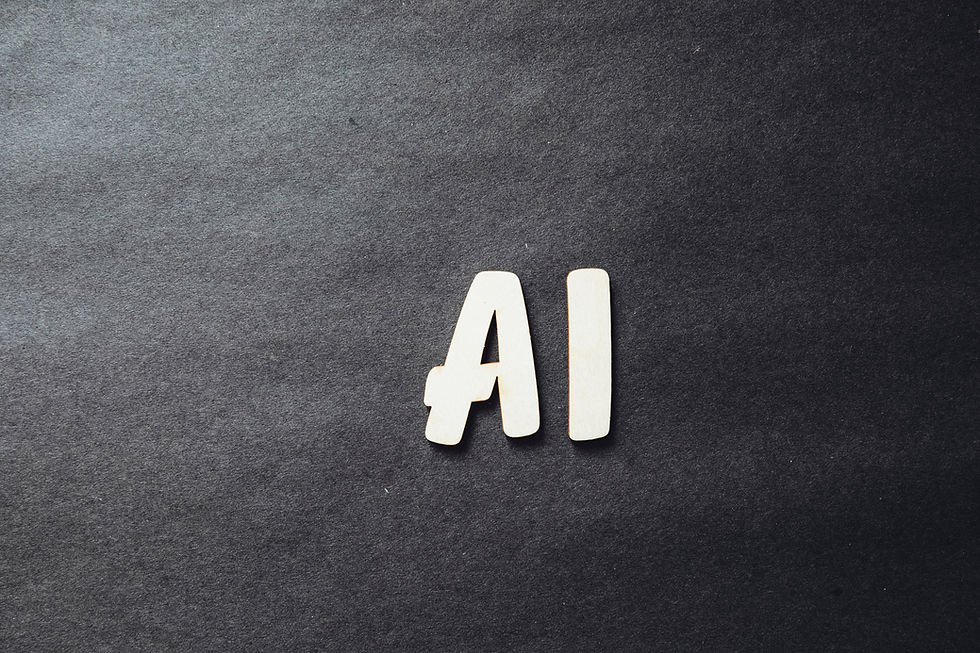
In complex interlinked plants, faults occur time and again that can influence the master machine. Often there are many small faults in an upstream or downstream machine, which can possibly affect the master machine. The plant operator is confronted with this problem on a daily basis. The disturbances provoke plant shutdowns, which considerably reduce the output of the plants.
Such disturbances cannot be analyzed easily and quickly. Data evaluation takes too long and ties up personnel.
For this purpose, the company Quantis has developed an algorithm that uses pattern recognition to identify all malfunctions of a plant and informs the responsible employee about them in time!
In times of automation and increasing digitalization in the beverage bottling industry, plant operators still have problems detecting plant shutdowns caused by undetected deterioration or malfunctions, despite all the progress that has been made.
These faults have a variety of causes and cannot always be detected immediately, because all machines in interlinked plants are subject to certain control behaviors. Therefore, for example, faults that occur in quick succession can affect the lead machine and bring the plant to a standstill. Changes in machine configurations can also cause different machine behavior, or there can be an increase in intrinsic faults due to outdated machines. A simple lack of materials can also be the reason for a plant shutdown.
In the event of a plant breakdown, a quick analysis of the cause is often not possible without problems. Visually, it is possible to identify occurring malfunctions, but the evaluation takes some time. This results in costs that must be avoided. The plant management's specifications regarding output are tightly set, and may not be achievable at the end of the day.
The algorithm developed by Quantis uses pattern recognition to identify all faults in the plant in near real time!
The algorithm permanently measures the data streams from the machines of a plant and evaluates them. If a malfunction or deterioration has been detected on one of the machines, the algorithm categorizes and prioritizes these malfunctions according to criticality, because not every malfunction reaches the lead machine. In case of creeping deterioration or changes in the behavior of the plant, the algorithm sends an SMS or e-mail to the person in charge, thus preventing expensive plant breakdowns, because regulating measures can be taken in time before machine downtimes occur! In addition, improvements in the behavior of the machines are also indicated in return, so it can be read that the initiated measures are also effective. The algorithm also makes tendency statements about future behavior of the machines in a plant, and informs the responsible person already at the right time, if there is a deterioration.
This process is already being used successfully by customers.
No long learning process is required for the algorithm, the time span between implementation and the correct predictions is weeks, not months! Quantis is far ahead of generating digital twins with its technology, as this effort is much larger, more time-consuming and thus considerably more expensive for the plant operator.
The data obtained from the algorithm is presented clearly and in graphically prepared dashboards. Heatmaps and histograms are valuable tools to show not only short-term trends, but also long-term changes in the behavior of individual machines. The results presented do not require any complicated analysis or interpretation by personnel; the data is presented in an easy-to-understand manner.
The use of the algorithm can be applied in different types of lines in the filling industry, such as PET, glass or canning lines.
The algorithm can also be integrated into existing IIoT platforms or other MES solutions. Quantis also offers its own platform solution, the Pocket Factory, to implement the algorithm at customers' sites, according to their needs.
For plant operators, it is important for the algorithm to
monitor creeping changes in behavior and malfunctions of the individual machines in a plant
send notifications in case of deterioration of the plant
easily readable results without analysis and interpretation
to be used for different types of equipment
enable a 50% reduction in plant downtime caused by faults
integrate with other IIoT platforms and MES solutions
be already successfully in use.
We promise that it works!
Picture by Unsplash Photo Markus Spiske
Commentaires